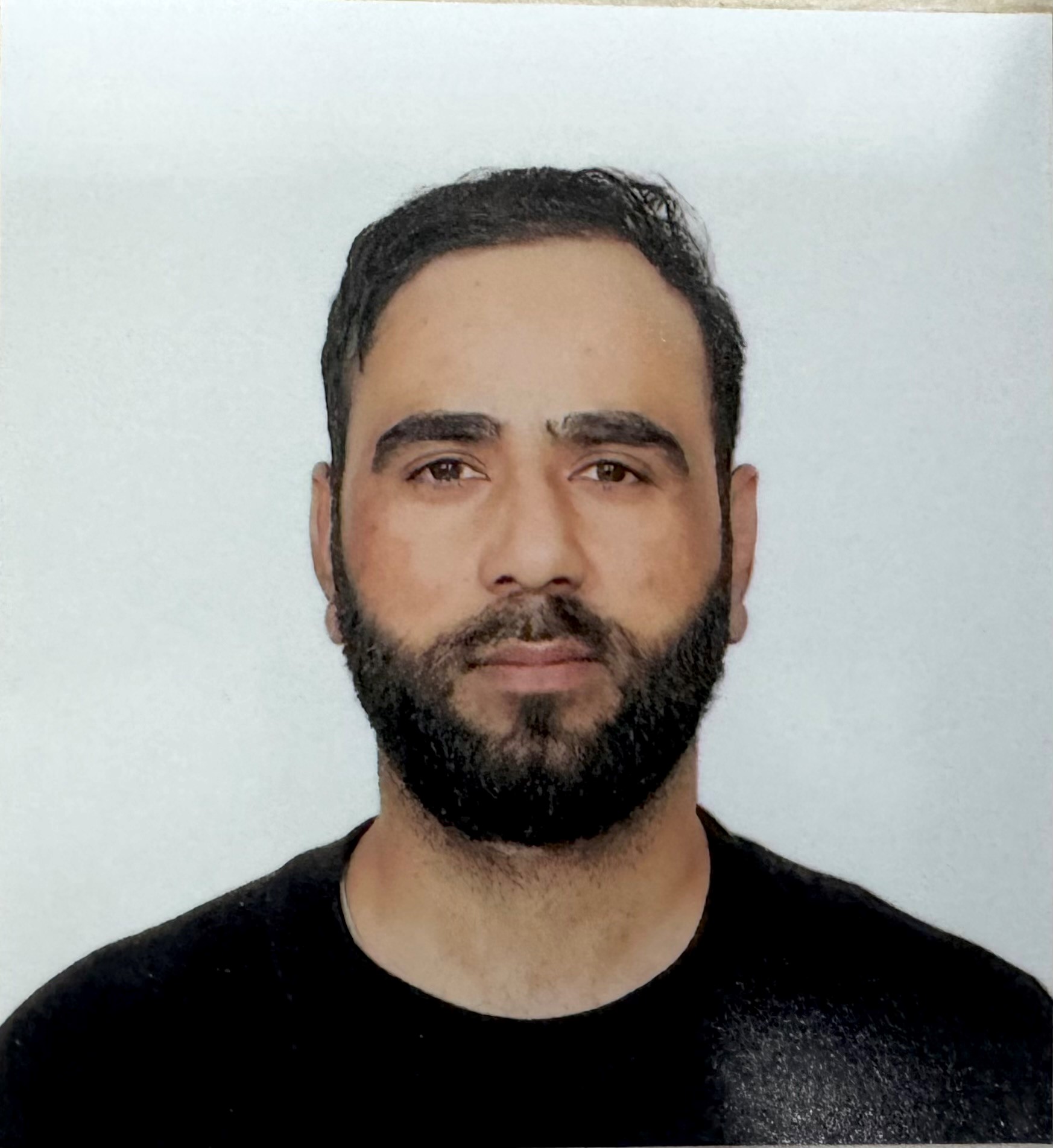
Novel phenotyping features using YOLOv8 deep learning architecture to segment stomatal images and accurately capture geometric properties
Sheikh Mansoor1,2, Thanh Tuan Thai2, Yong Suk Chung2, Kyung‑Hwan Boo1*
1Subtropical/Tropical Organism Gene Bank, Jeju National University, Jeju 63243, Republic of Korea
2Department of Plant Resources and Environment, Jeju National University, Jeju 63243, Republic of Korea
Stomata, tiny pores found on plant leaves, are pivotal in controlling the exchange of gases and the release of water vapor, significantly impacting photosynthesis and transpiration. Analyzing stomatal features such as their size, density, and arrangement is crucial for comprehending plant physiology, their ability to adapt to different environments, and climate changes. This investigation strategically employs the refined YOLOv8 model, a complex deep learning framework, to address the challenging task of instance segmenting stomata. The dataset, comprising 1260 images annotated for both stomatal pores and guard cells, was preprocessed and split into training and validation sets with an 85:15 ratio. Our new approach showed outstanding accuracy in predicting stomatal parameters such as area, width, length, and orientation. This underscores the effectiveness of incorporating deep learning for precise and efficient analysis of stomata on a large scale. The introduction of a novel opening ratio, which measures the area of the pore relative to the area of the accompanying stomatal guard cells, represents a major advance in analyzing stomatal behavior. This study has profound implications in precise measurement and analysis of stomatal traits and offers new opportunities for comprehending how plants respond to environmental stimuli, thereby improving monitoring capabilities.